
Experimental Tools to Diagnose Autism
Experimental Tools to Diagnose Autism
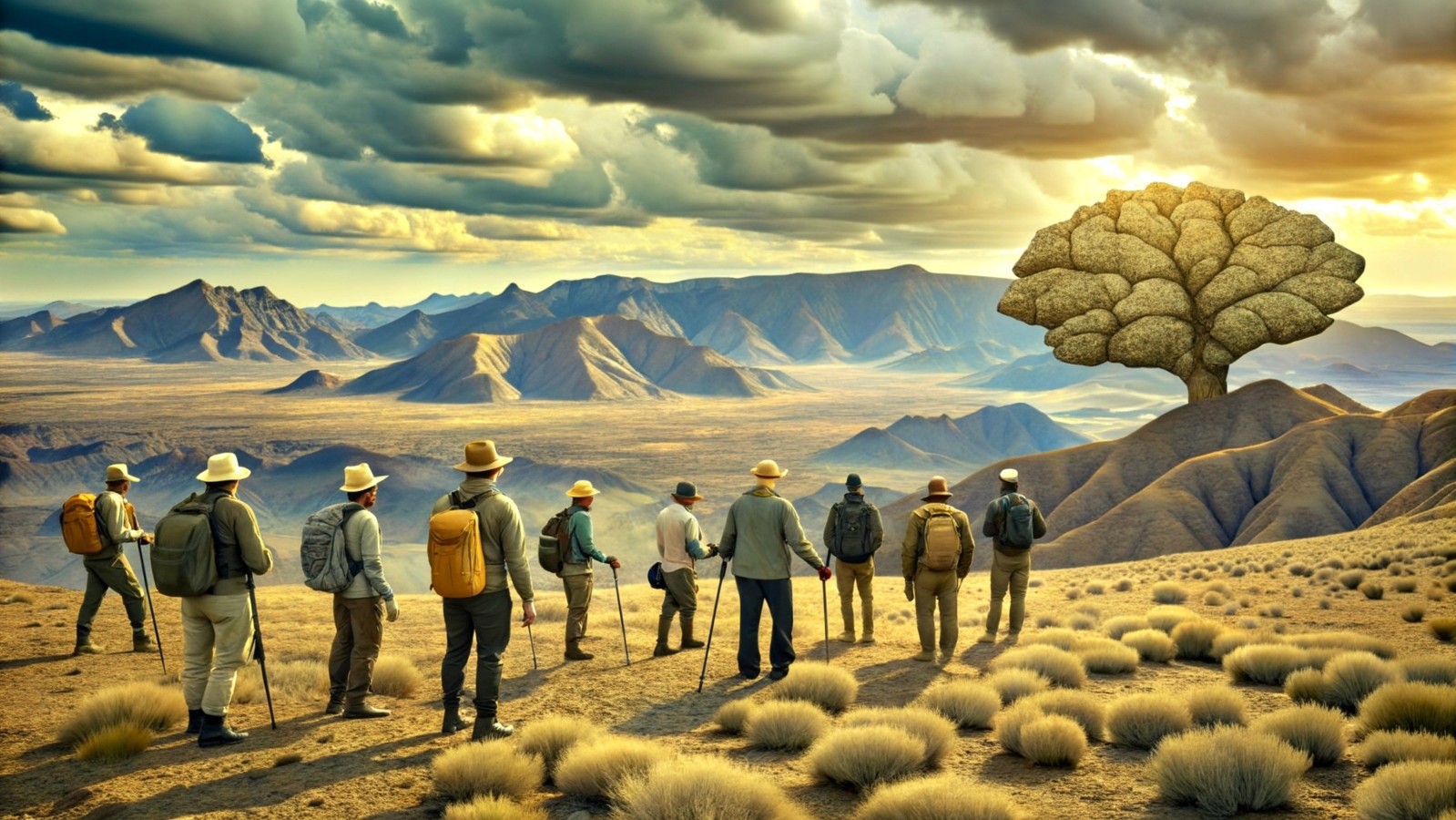
Explanation
upd
1/29/24
Precisely
Simpler
Shorter
Main Thing
Experimental tools to diagnose autism are innovative methods under investigation. These tools are part of ongoing research to improve the accuracy and timing of autism diagnosis. They are not yet standard practice but could offer new insights.
Spinal Fluid Analysis: Dr. Karen Parker's research involves studying cerebrospinal fluid for biomarkers of autism.
Eye Tracking Technology: This tool measures gaze patterns that may differ in individuals with autism.
Machine Learning Algorithms: These analyze behavioral or genetic data to identify autism-related patterns.
Brain Imaging Techniques: Methods like fMRI may show unique brain communication patterns in autism.
Each of these tools is in various stages of research and testing. For the latest results, one should consult scientific journals or research institutions' publications.
In real life, a child might be part of a study where these experimental tools are used to gather data and refine the methods of early autism diagnosis.
Terms
Autism: A developmental disorder characterized by difficulties with social interaction and communication, and by restricted and repetitive behavior. Example: A child with autism might have trouble making eye contact and engage in repetitive behaviors like lining up toys.
Spinal Fluid: Also known as cerebrospinal fluid, it is a clear, colorless body fluid found in the brain and spinal cord. It is believed to contain clues about brain health and function. Example: In Dr. Karen Parker's research, spinal fluid is analyzed to find potential biomarkers of autism.
Machine Learning: A type of artificial intelligence that enables computers to learn from and make decisions or predictions based on data. Example: In autism research, machine learning algorithms might analyze behavioral data to predict autism.
Brain Imaging: Techniques like fMRI that show how different areas of the brain communicate. Example: A research study on autism might use fMRI to look for unique brain communication patterns in participants with autism.
An Analogy
Think of these experimental tools like explorers charting new territory. Just as explorers use different tools like compasses, maps, and telescopes to understand new lands, researchers use these experimental tools to uncover new insights about autism.
A Main Misconception
A common misconception is that autism can be diagnosed with a single test. In reality, autism diagnosis is complex and often involves multiple tools and assessments. Experimental tools like those mentioned here are part of ongoing research to improve this process.
The History
1940s: Autism is first identified as a distinct condition by Leo Kanner. At this time, diagnosis is based purely on behavioral observations.
1980s: Structured diagnostic interviews and observational scales become the standard tools for diagnosing autism. The Autism Diagnostic Interview (ADI) and later the Autism Diagnostic Observation Schedule (ADOS) are developed during this period.
2000s: Genetic testing begins to be used to identify potential genetic causes of autism, adding a new tool to the diagnostic process.
2010s and Beyond: New experimental tools begin to emerge, including eye tracking technology, machine learning algorithms, and spinal fluid analysis. These tools are still in the research phase but hold promise for improving early detection and understanding of autism.
Three Cases How to Use It Right Now
Parental Observation: As a parent, understanding these experimental tools can help you observe your child's behavior with a more informed perspective. For example, you might notice patterns in your child's gaze or social interactions that you want to discuss with a healthcare provider.
Advocacy: Knowledge of these experimental tools can help you advocate for more research funding or better resources for individuals with autism in your community.
Education: If you're a teacher or work with children, understanding these tools can help you better support students who might be on the autism spectrum and communicate effectively with their parents or caregivers.
Interesting Facts
Autism is a spectrum disorder, meaning symptoms can vary widely from person to person.
There is no known single cause of autism, but both genetics and environment are believed to play a role. Experimental tools like genetic testing and brain imaging are helping researchers explore these factors.
Early diagnosis of autism, which these experimental tools aim to facilitate, can significantly improve outcomes for individuals with autism.
Experimental tools for autism diagnosis, such as spinal fluid analysis and machine learning algorithms, are part of ongoing research to improve the accuracy and timing of diagnosis.
The use of machine learning in autism diagnosis is a relatively new development, but early results show promise in identifying patterns in large datasets that might predict autism.
Main Thing
Experimental tools to diagnose autism are innovative methods under investigation. These tools are part of ongoing research to improve the accuracy and timing of autism diagnosis. They are not yet standard practice but could offer new insights.
Spinal Fluid Analysis: Dr. Karen Parker's research involves studying cerebrospinal fluid for biomarkers of autism.
Eye Tracking Technology: This tool measures gaze patterns that may differ in individuals with autism.
Machine Learning Algorithms: These analyze behavioral or genetic data to identify autism-related patterns.
Brain Imaging Techniques: Methods like fMRI may show unique brain communication patterns in autism.
Each of these tools is in various stages of research and testing. For the latest results, one should consult scientific journals or research institutions' publications.
In real life, a child might be part of a study where these experimental tools are used to gather data and refine the methods of early autism diagnosis.
Terms
Autism: A developmental disorder characterized by difficulties with social interaction and communication, and by restricted and repetitive behavior. Example: A child with autism might have trouble making eye contact and engage in repetitive behaviors like lining up toys.
Spinal Fluid: Also known as cerebrospinal fluid, it is a clear, colorless body fluid found in the brain and spinal cord. It is believed to contain clues about brain health and function. Example: In Dr. Karen Parker's research, spinal fluid is analyzed to find potential biomarkers of autism.
Machine Learning: A type of artificial intelligence that enables computers to learn from and make decisions or predictions based on data. Example: In autism research, machine learning algorithms might analyze behavioral data to predict autism.
Brain Imaging: Techniques like fMRI that show how different areas of the brain communicate. Example: A research study on autism might use fMRI to look for unique brain communication patterns in participants with autism.
An Analogy
Think of these experimental tools like explorers charting new territory. Just as explorers use different tools like compasses, maps, and telescopes to understand new lands, researchers use these experimental tools to uncover new insights about autism.
A Main Misconception
A common misconception is that autism can be diagnosed with a single test. In reality, autism diagnosis is complex and often involves multiple tools and assessments. Experimental tools like those mentioned here are part of ongoing research to improve this process.
The History
1940s: Autism is first identified as a distinct condition by Leo Kanner. At this time, diagnosis is based purely on behavioral observations.
1980s: Structured diagnostic interviews and observational scales become the standard tools for diagnosing autism. The Autism Diagnostic Interview (ADI) and later the Autism Diagnostic Observation Schedule (ADOS) are developed during this period.
2000s: Genetic testing begins to be used to identify potential genetic causes of autism, adding a new tool to the diagnostic process.
2010s and Beyond: New experimental tools begin to emerge, including eye tracking technology, machine learning algorithms, and spinal fluid analysis. These tools are still in the research phase but hold promise for improving early detection and understanding of autism.
Three Cases How to Use It Right Now
Parental Observation: As a parent, understanding these experimental tools can help you observe your child's behavior with a more informed perspective. For example, you might notice patterns in your child's gaze or social interactions that you want to discuss with a healthcare provider.
Advocacy: Knowledge of these experimental tools can help you advocate for more research funding or better resources for individuals with autism in your community.
Education: If you're a teacher or work with children, understanding these tools can help you better support students who might be on the autism spectrum and communicate effectively with their parents or caregivers.
Interesting Facts
Autism is a spectrum disorder, meaning symptoms can vary widely from person to person.
There is no known single cause of autism, but both genetics and environment are believed to play a role. Experimental tools like genetic testing and brain imaging are helping researchers explore these factors.
Early diagnosis of autism, which these experimental tools aim to facilitate, can significantly improve outcomes for individuals with autism.
Experimental tools for autism diagnosis, such as spinal fluid analysis and machine learning algorithms, are part of ongoing research to improve the accuracy and timing of diagnosis.
The use of machine learning in autism diagnosis is a relatively new development, but early results show promise in identifying patterns in large datasets that might predict autism.
Main Thing
Experimental tools to diagnose autism are innovative methods under investigation. These tools are part of ongoing research to improve the accuracy and timing of autism diagnosis. They are not yet standard practice but could offer new insights.
Spinal Fluid Analysis: Dr. Karen Parker's research involves studying cerebrospinal fluid for biomarkers of autism.
Eye Tracking Technology: This tool measures gaze patterns that may differ in individuals with autism.
Machine Learning Algorithms: These analyze behavioral or genetic data to identify autism-related patterns.
Brain Imaging Techniques: Methods like fMRI may show unique brain communication patterns in autism.
Each of these tools is in various stages of research and testing. For the latest results, one should consult scientific journals or research institutions' publications.
In real life, a child might be part of a study where these experimental tools are used to gather data and refine the methods of early autism diagnosis.
Terms
Autism: A developmental disorder characterized by difficulties with social interaction and communication, and by restricted and repetitive behavior. Example: A child with autism might have trouble making eye contact and engage in repetitive behaviors like lining up toys.
Spinal Fluid: Also known as cerebrospinal fluid, it is a clear, colorless body fluid found in the brain and spinal cord. It is believed to contain clues about brain health and function. Example: In Dr. Karen Parker's research, spinal fluid is analyzed to find potential biomarkers of autism.
Machine Learning: A type of artificial intelligence that enables computers to learn from and make decisions or predictions based on data. Example: In autism research, machine learning algorithms might analyze behavioral data to predict autism.
Brain Imaging: Techniques like fMRI that show how different areas of the brain communicate. Example: A research study on autism might use fMRI to look for unique brain communication patterns in participants with autism.
An Analogy
Think of these experimental tools like explorers charting new territory. Just as explorers use different tools like compasses, maps, and telescopes to understand new lands, researchers use these experimental tools to uncover new insights about autism.
A Main Misconception
A common misconception is that autism can be diagnosed with a single test. In reality, autism diagnosis is complex and often involves multiple tools and assessments. Experimental tools like those mentioned here are part of ongoing research to improve this process.
The History
1940s: Autism is first identified as a distinct condition by Leo Kanner. At this time, diagnosis is based purely on behavioral observations.
1980s: Structured diagnostic interviews and observational scales become the standard tools for diagnosing autism. The Autism Diagnostic Interview (ADI) and later the Autism Diagnostic Observation Schedule (ADOS) are developed during this period.
2000s: Genetic testing begins to be used to identify potential genetic causes of autism, adding a new tool to the diagnostic process.
2010s and Beyond: New experimental tools begin to emerge, including eye tracking technology, machine learning algorithms, and spinal fluid analysis. These tools are still in the research phase but hold promise for improving early detection and understanding of autism.
Three Cases How to Use It Right Now
Parental Observation: As a parent, understanding these experimental tools can help you observe your child's behavior with a more informed perspective. For example, you might notice patterns in your child's gaze or social interactions that you want to discuss with a healthcare provider.
Advocacy: Knowledge of these experimental tools can help you advocate for more research funding or better resources for individuals with autism in your community.
Education: If you're a teacher or work with children, understanding these tools can help you better support students who might be on the autism spectrum and communicate effectively with their parents or caregivers.
Interesting Facts
Autism is a spectrum disorder, meaning symptoms can vary widely from person to person.
There is no known single cause of autism, but both genetics and environment are believed to play a role. Experimental tools like genetic testing and brain imaging are helping researchers explore these factors.
Early diagnosis of autism, which these experimental tools aim to facilitate, can significantly improve outcomes for individuals with autism.
Experimental tools for autism diagnosis, such as spinal fluid analysis and machine learning algorithms, are part of ongoing research to improve the accuracy and timing of diagnosis.
The use of machine learning in autism diagnosis is a relatively new development, but early results show promise in identifying patterns in large datasets that might predict autism.
Materials for self-study
11
Zhuowen Yin, Xinyao Ding, Xin Zhang, Zhengwang Wu, Li Wang, Xiangmin Xu, Gang Li @Cerebral Cortex
5/2/24
8
Dr. Karen Parker, Andrew Huberman
12/11/23
11
Zhuowen Yin, Xinyao Ding, Xin Zhang, Zhengwang Wu, Li Wang, Xiangmin Xu, Gang Li @Cerebral Cortex
5/2/24
8
Dr. Karen Parker, Andrew Huberman
12/11/23
11
Zhuowen Yin, Xinyao Ding, Xin Zhang, Zhengwang Wu, Li Wang, Xiangmin Xu, Gang Li @Cerebral Cortex
5/2/24
8
Dr. Karen Parker, Andrew Huberman
12/11/23
+ Suggest a material
Register to Use the Bookmarking Feature
By registering, you can:
Save materials for later (bookmarks)
Track your progress on roadmaps and blocks
Access selected medium and full roadmaps for free
Get notified about new roadmaps
Register to Use the Bookmarking Feature
By registering, you can:
Save materials for later (bookmarks)
Track your progress on roadmaps and blocks
Access selected medium and full roadmaps for free
Get notified about new roadmaps
Register to Use the Bookmarking Feature
By registering, you can:
Save materials for later (bookmarks)
Track your progress on roadmaps and blocks
Access selected medium and full roadmaps for free
Get notified about new roadmaps
Check exercise
You read a news article about a new experimental tool for diagnosing autism that uses a machine learning algorithm. What questions might you ask to understand more about how this tool works?
Register to Track Your Progress
By registering, you can:
Save materials for later (bookmarks)
Track your progress on roadmaps and blocks
Access selected medium and full roadmaps for free
Get notified about new roadmaps
Register to Track Your Progress
By registering, you can:
Save materials for later (bookmarks)
Track your progress on roadmaps and blocks
Access selected medium and full roadmaps for free
Get notified about new roadmaps
Register to Track Your Progress
By registering, you can:
Save materials for later (bookmarks)
Track your progress on roadmaps and blocks
Access selected medium and full roadmaps for free
Get notified about new roadmaps
Updates
No updates yet.
11
5/2/24
A new deep learning method significantly improves early autism diagnosis using structural MR images.
Zhuowen Yin, Xinyao Ding, Xin Zhang, Zhengwang Wu, Li Wang, Xiangmin Xu, Gang Li @Cerebral Cortex
45
12/11/23
The study demonstrates that several machine learning algorithms can effectively diagnose ASD in toddlers based on gaze behavior.
Fernandez-Lanvin Daniel, Gonzalez-Rodriguez Martin, De-Andres Javier, Camero Raquel @Springer
No updates yet.
11
5/2/24
A new deep learning method significantly improves early autism diagnosis using structural MR images.
Zhuowen Yin, Xinyao Ding, Xin Zhang, Zhengwang Wu, Li Wang, Xiangmin Xu, Gang Li @Cerebral Cortex
45
12/11/23
The study demonstrates that several machine learning algorithms can effectively diagnose ASD in toddlers based on gaze behavior.
Fernandez-Lanvin Daniel, Gonzalez-Rodriguez Martin, De-Andres Javier, Camero Raquel @Springer
No updates yet.
11
5/2/24
A new deep learning method significantly improves early autism diagnosis using structural MR images.
Zhuowen Yin, Xinyao Ding, Xin Zhang, Zhengwang Wu, Li Wang, Xiangmin Xu, Gang Li @Cerebral Cortex
45
12/11/23
The study demonstrates that several machine learning algorithms can effectively diagnose ASD in toddlers based on gaze behavior.
Fernandez-Lanvin Daniel, Gonzalez-Rodriguez Martin, De-Andres Javier, Camero Raquel @Springer
Roadmaps where it's used
Related blocks
Share